Understanding the Four Principles of Explainable AI
Understanding the Four Principles of Explainable AI
Andrea Harston
1/26/20252 min read
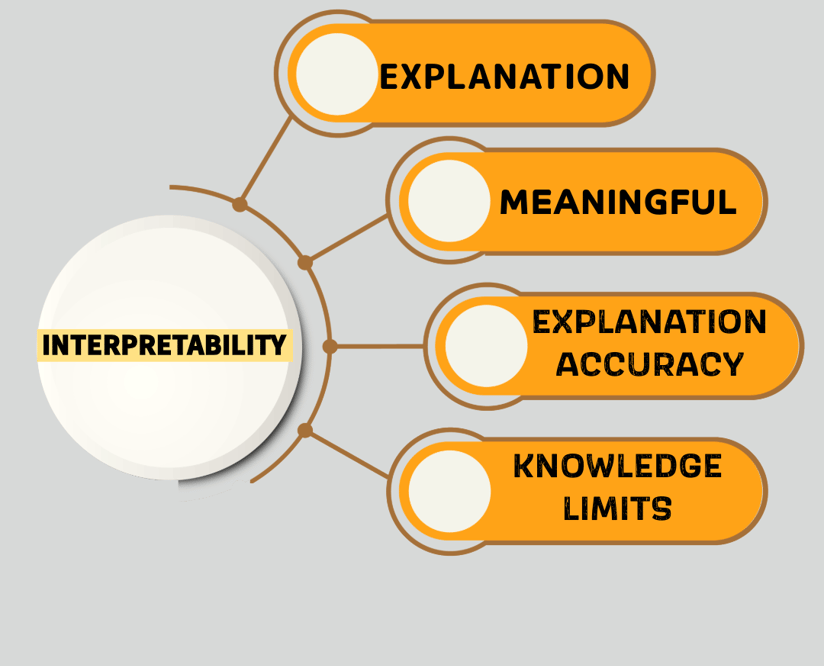
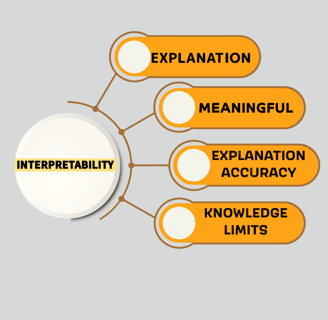
As AI systems become increasingly sophisticated and integrated into critical decision-making processes across various sectors, the need for transparency and accountability has become paramount. Many AI models operate in a “Black Box.” Their inner workings are obscured and difficult to understand, raising concerns about bias, fairness, and the potential for unintended consequences. Explainable AI (XAI) emerges as a critical solution to these challenges, aiming to make AI systems more transparent and understandable to humans.
The National Institute of Standards and Technology (NIST) has taken a leading role in establishing a framework for explainable AI (XAI). Recognizing the critical need for transparency and trust in AI systems, NIST has identified four core principles that guide the development and deployment of responsible AI. These principles provide a valuable framework for researchers, developers, and organizations to ensure that AI systems are not only effective but also understandable, trustworthy, and beneficial to society.
The Four Core Principles
1. Explanation:
The Foundation: AI systems should be able to provide clear and concise explanations for their decisions and outputs. This could involve:
Showing the data points that influenced a particular prediction.
Visualizing the decision-making process.
Providing a step-by-step breakdown of the logic used by the AI.
2. Meaningful:
Human-Centered: Explanations should be understandable to the intended audience, whether it's a data scientist, a business leader, or an everyday user.
Relevant: The explanations should address the user's specific needs and questions. For example, a doctor might need to understand why an AI system recommended a particular treatment, while a loan officer might need to understand why a loan application was denied.
3. Explanation Accuracy:
Truthful and Reliable: The explanations provided by the AI system must accurately reflect the reasoning behind its decisions.
Free from Bias: Explanations should not be misleading or biased, and they should accurately represent the true factors that influenced the AI's output.
4. Knowledge Limits:
Awareness of Limitations: AI systems should be aware of their own limitations and only operate within the scope of their intended use.
Confidence Intervals: AI systems should be able to communicate their level of confidence in their predictions. For example, if an AI system is uncertain about a particular decision, it should be able to indicate that uncertainty to the user.
Why are these principles important?
Increased Trust: By making AI systems more transparent and understandable, we can increase trust in their decisions. This is crucial for widespread adoption in critical areas like healthcare, finance, and criminal justice.
Improved Decision-Making: Explainable AI empowers users to understand and critically evaluate the decisions made by AI systems, leading to more informed and responsible decision-making.
Enhanced Accountability: When AI systems can explain their reasoning, it becomes easier to identify and rectify biases, errors, and other issues.
Regulatory Compliance: In many industries, regulations are emerging that require AI systems to be explainable and transparent.
Conclusion
The development of explainable AI is crucial for ensuring the responsible and ethical development and deployment of AI technologies. By adhering to these four principles, we can build AI systems that are not only powerful but also trustworthy and beneficial to society.